Github: https://github.com/SuminHan/Traffic-UAGCRNTF
We share our code for deep-learning research from Sumin Han, Youngjun Park, Minji Lee, Jisun An, and Dongman Lee, “Enhancing Spatio-temporal Traffic Prediction through Urban Human Activity Analysis,” has been accepted to 32nd ACM International Conference on Information and Knowledge Management Paper Code
This code contains not only our proposed UAGCRN and UAGCTransformer, but also DCRNN and GMAN which are baselines.
Model Architecture
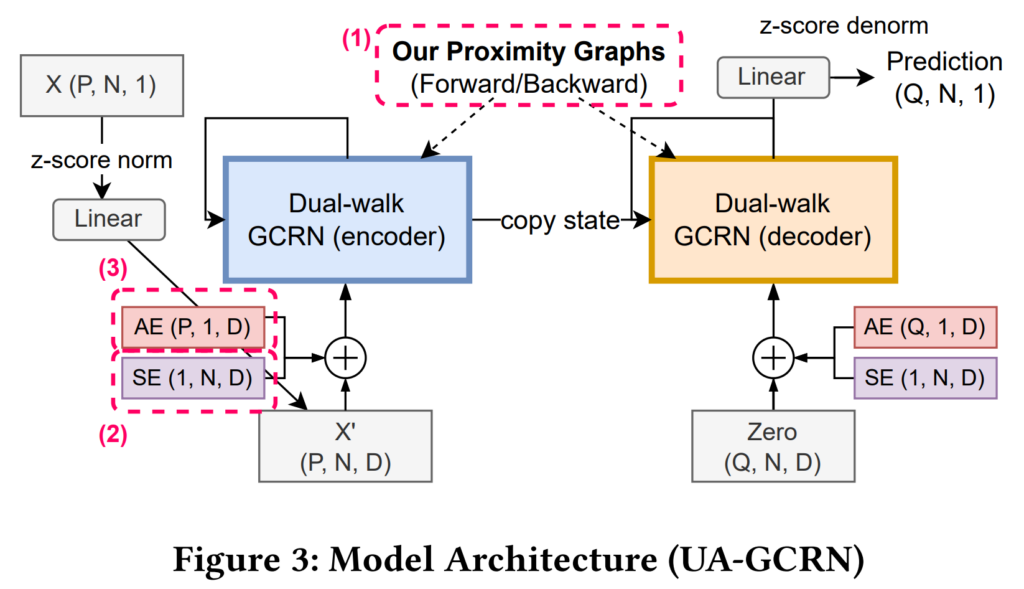
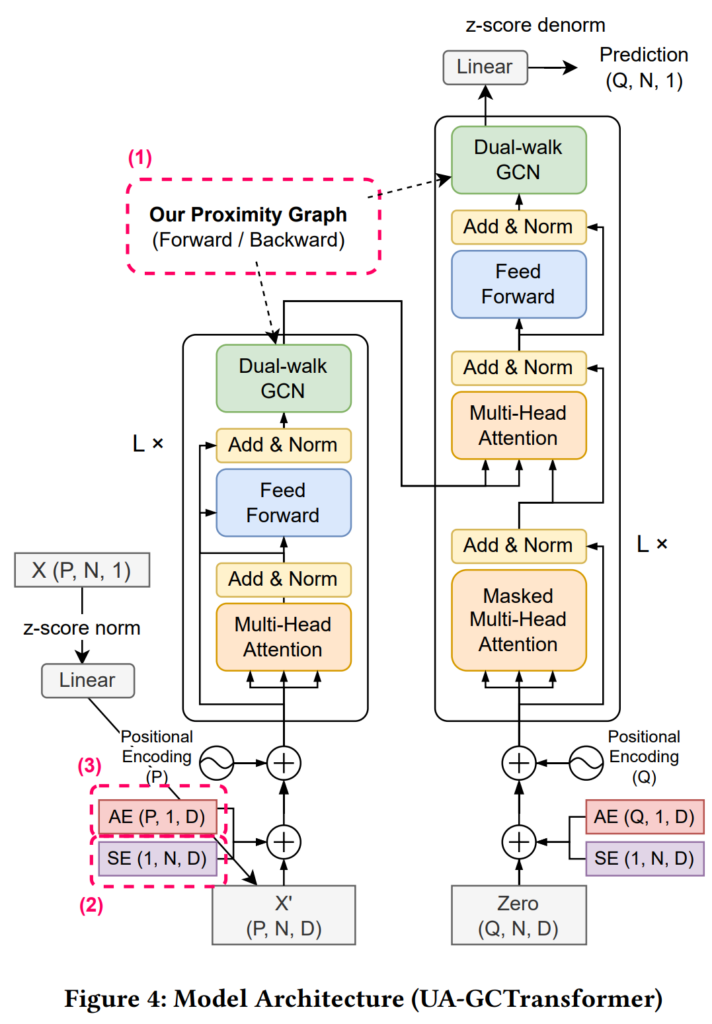
Code
This installation command worked with our code execution:
conda deactivate
conda env remove -n CIKM
conda create -n CIKM -y
conda activate CIKM
conda install python==3.10 -y
pip install tensorflow-gpu==2.10.0
pip install tqdm
pip install tables
pip install scipy==1.10.1
pip install pandas==1.5.3
pip install numpy==1.23.5
python train.py --model_name=MyUAGCRN --dataset=metr-la --Q=12 --activity_embedding --sensor_embedding --graph_type=cooccur_dist
python train.py --model_name=MyUAGCRN --dataset=pems-bay --Q=12 --activity_embedding --sensor_embedding --graph_type=cooccur_dist
python train.py --model_name=MyUAGCRN --dataset=pemsd7 --Q=9 --activity_embedding --sensor_embedding --graph_type=cooccur_dist
python train.py --model_name=MyUAGCTransformer --dataset=metr-la --Q=12 --activity_embedding --sensor_embedding --graph_type=cooccur_dist
python train.py --model_name=MyUAGCTransformer --dataset=pems-bay --Q=12 --activity_embedding --sensor_embedding --graph_type=cooccur_dist
python train.py --model_name=MyUAGCTransformer --dataset=pemsd7 --Q=9 --activity_embedding --sensor_embedding --graph_type=cooccur_dist
Dataset
Traffic Dataset
- california-vds.json: https://pems.dot.ca.gov/
- metr-la, pems-bay: DCRNN, https://github.com/liyaguang/DCRNN
- pemsd7: STGCN, https://github.com/VeritasYin/STGCN_IJCAI-18
Traffic Dataset Description
DATASET | N | Speed (miles/hour) | Datasize | PLACE | DURATION | INTERVAL |
---|---|---|---|---|---|---|
METR_LA | 207 | 54 ± 20 | 34,249 | Los Angeles, USA | Mar. 1, 2012 – Jun. 27, 2012 | 5min |
PEMS_BAY | 325 | 62 ± 10 | 52,093 | San Francisco Bay Area, USA | Jan. 1, 2017 – Jun. 30, 2017 | 5min |
PEMSD7 | 228 | 59 ± 13 | 12,652 | Los Angeles, USA | May. 1, 2012 – Jun.30, 2012 | 5min |
National Household Survey
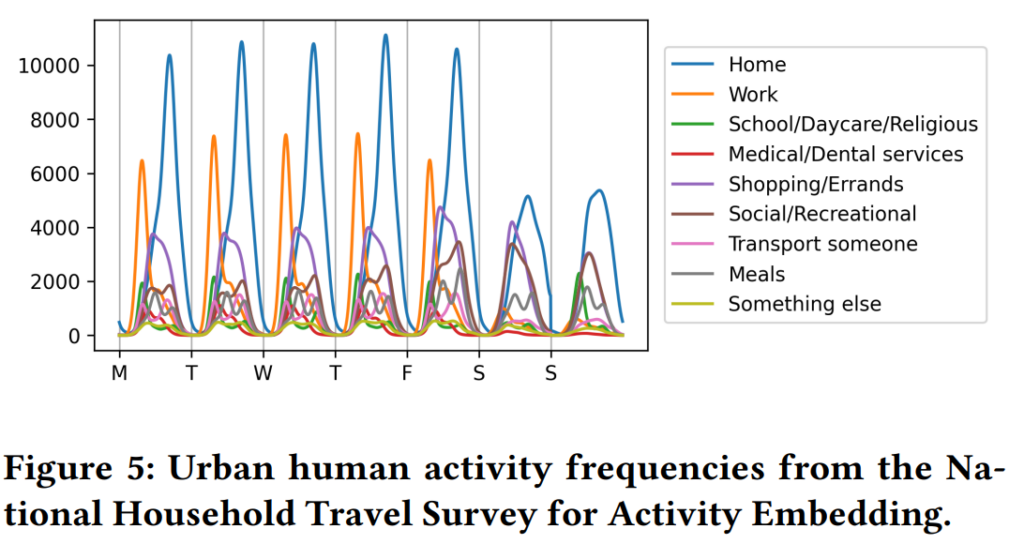
Citation
To recognize the valuable role of National Household Travel Survey (NHTS) data in the transportation research process and to facilitate repeatability of the research, users of NHTS data are asked to formally acknowledge the data source. Where possible, this acknowledgement should take place in the form of a formal citation, such as when writing a research report, planning document, on-line article, and other publications. The citation can be formatted as follows: U.S. Department of Transportation, Federal Highway Administration, 2017 National Household Travel Survey. URL: http://nhts.ornl.gov.
Download page
- https://nhts.ornl.gov/downloads
- survey data csv link: https://nhts.ornl.gov/assets/2016/download/csv.zip
Sensor Location Correction
Corrected sensor location with osm path files are found in
- METR-LA: dataset/corrected-metr-la-sensorid-osm-path-uv.csv
- PEMS-BAY: dataset/corrected-pemsbay-sensorid-osm-path-uv.csv
- PEMSD7: dataset/corrected-pemsd7-sensorid-osm-path-uv.csv
METR-LA

PEMS-BAY

PEMSD7

Performance Comparison

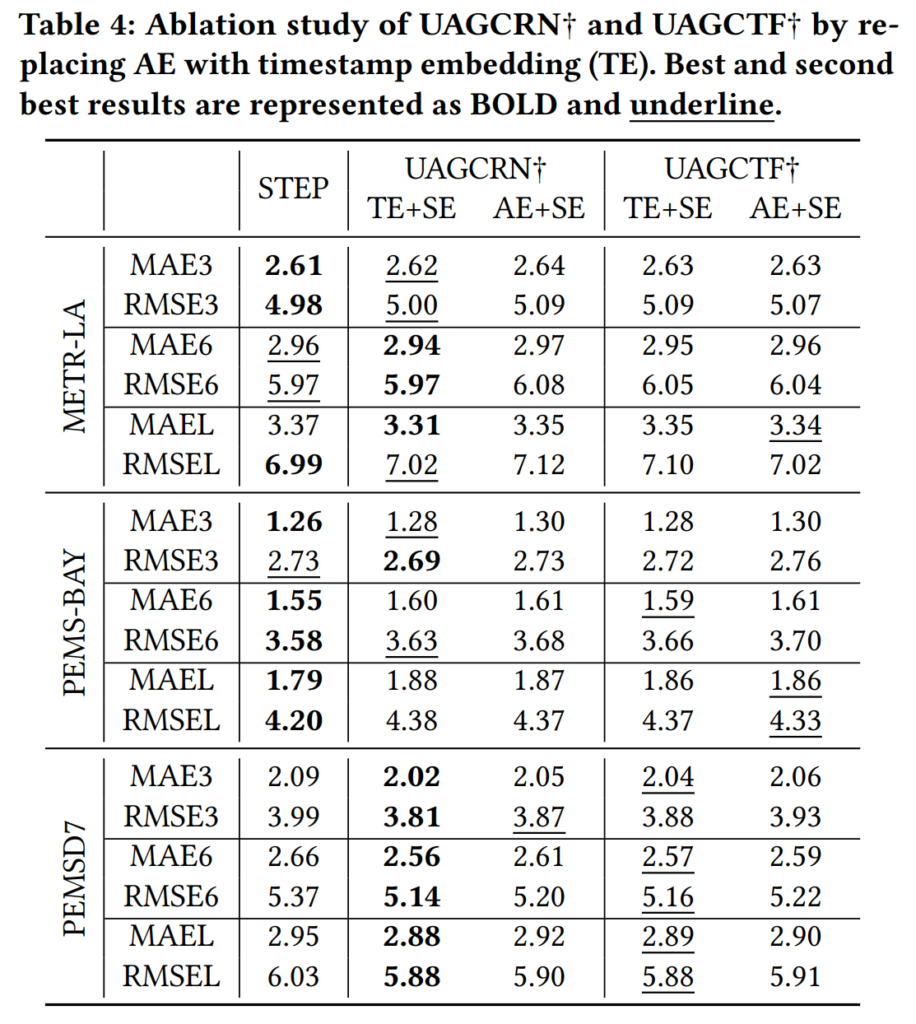
Ablation Study
